EPRI ADMIN (talk | contribs) |
EPRI ADMIN (talk | contribs) |
||
(5 intermediate revisions by the same user not shown) | |||
Line 22: | Line 22: | ||
Even beyond this issue, however, wind and solar may also impact on resource adequacy studies as their variability and uncertainty may cause periods when, even though sufficient capacity may be available, there is insufficient flexibility. This provides the motivation for much of the studies in this guidelines document, where flexibility sufficiency needs to be assessed to ensure that situations do not occur more often than desired where, even though installed capacity is sufficient, ramping capability is lacking. As described before, this then requires more detailed consideration of system operations in the resource planning timeframe. | Even beyond this issue, however, wind and solar may also impact on resource adequacy studies as their variability and uncertainty may cause periods when, even though sufficient capacity may be available, there is insufficient flexibility. This provides the motivation for much of the studies in this guidelines document, where flexibility sufficiency needs to be assessed to ensure that situations do not occur more often than desired where, even though installed capacity is sufficient, ramping capability is lacking. As described before, this then requires more detailed consideration of system operations in the resource planning timeframe. | ||
− | As an example of this additional detail that is required, in 2013 EPRI studied the issue of resource adequacy with high levels of renewables using the SERVM tool, as run by Astrape Consulting | + | As an example of this additional detail that is required, in 2013 EPRI studied the issue of resource adequacy with high levels of renewables using the SERVM tool, as run by Astrape Consulting <ref name="F11">Power System Flexibility Metrics EPRI, Palo Alto, CA: 2013. 3002000331</ref>. This is a probabilistic production cost tool, using thousands of annual production cost runs to identify loss of load expectation (LOLE) and similar metrics. For the 2013 study, the short term uncertainty (day ahead, hour ahead, etc.) associated with wind and solar power was included in the model, and the impact of this on the loss of load was determined. This was done for a high solar and wind penetration case in California. As shown in Figure 2‑2, this resulted in additional loss of load compared to a case where renewables were assumed to be forecasted perfectly. This shows that if uncertainty is not considered in the model, the LOLE may be underestimated by 0.75 days per year (in that particular study), which could result in a system not able to meet the required standards, or that may require additional capacity. |
− | [[File:faimage7.png|center|border|frame|Figure ‑ Impact of uncertainty on resource adequacy, from previous EPRI work | + | [[File:faimage7.png|center|border|frame|Figure ‑ Impact of uncertainty on resource adequacy, from previous EPRI work <ref name="F11"></ref>]] |
While it is not clear how this should impact on planning standards (as discussed later in the summary of the CES-21 project), and whether the same LOLE standard should be used to ensure sufficient flexibility as used for capacity (i.e. whether one would want to still maintain a loss of load of 1 day in ten years, even when adding consideration of ramping into the calculation), it is nonetheless clear that the variability and uncertainty of renewables has the potential to impact on resource adequacy, leading to increased need to consider these issues. The SERVM tool is discussed in numerous places later, in particular for discussion of the CES-21 project in California, where it was used to investigate the need for flexibility metrics to assist planning processes. | While it is not clear how this should impact on planning standards (as discussed later in the summary of the CES-21 project), and whether the same LOLE standard should be used to ensure sufficient flexibility as used for capacity (i.e. whether one would want to still maintain a loss of load of 1 day in ten years, even when adding consideration of ramping into the calculation), it is nonetheless clear that the variability and uncertainty of renewables has the potential to impact on resource adequacy, leading to increased need to consider these issues. The SERVM tool is discussed in numerous places later, in particular for discussion of the CES-21 project in California, where it was used to investigate the need for flexibility metrics to assist planning processes. | ||
Line 58: | Line 58: | ||
For the past several years, EPRI has performed work in the project, “Strategic and Flexible Planning” on the topic of operational flexibility. This was part of the Grid Planning (P40) program from 2011 until 2014, and from 2015 moved to be part of the Bulk System Integration of Variable Generation (P173) program. The focus has been on understanding the need for and provision of operational flexibility in power system planning, focusing on a number of aspects, as summarized in Figure 2‑4: | For the past several years, EPRI has performed work in the project, “Strategic and Flexible Planning” on the topic of operational flexibility. This was part of the Grid Planning (P40) program from 2011 until 2014, and from 2015 moved to be part of the Bulk System Integration of Variable Generation (P173) program. The focus has been on understanding the need for and provision of operational flexibility in power system planning, focusing on a number of aspects, as summarized in Figure 2‑4: | ||
− | * Initial work used case studies to illustrate the need for flexibility and develop some initial concepts and a framework for assessment of the flexibility on the system | + | * Initial work used case studies to illustrate the need for flexibility and develop some initial concepts and a framework for assessment of the flexibility on the system <ref>Flexible and Strategic Transmission Planning. EPRI, Palo Alto, CA: 2011. 1021931</ref><ref>Metrics and Methods for Consideration of Flexibility in Power System Planning. EPRI, Palo Alto, CA: 2012. 1024274</ref><ref name="F11"></ref>. This included analyzing results from production cost tools to better understand flexibility issues, and introduced concepts such as consideration of different time horizons, use of different percentiles in assessing flexibility requirements, and the need to examine both upwards and downwards ramping. |
* Based on these initial studies, metrics and tools were developed to assess the needs for and provision of flexibility. This led to the creation of the InFLEXion tool, which has continued to improve over numerous versions. The latest version of InFLEXion, v5.1, is expected to be released in late 2018. It is likely that a user’s group will also be started in next few years. | * Based on these initial studies, metrics and tools were developed to assess the needs for and provision of flexibility. This led to the creation of the InFLEXion tool, which has continued to improve over numerous versions. The latest version of InFLEXion, v5.1, is expected to be released in late 2018. It is likely that a user’s group will also be started in next few years. | ||
− | * Resource adequacy and its links with flexibility has been explored, where the impact of variability and uncertainty on resource adequacy was outlined and demonstrated using a number of case studies. A specific case study on the impact of flexibility needs on resource adequacy was carried out in 2013 | + | * Resource adequacy and its links with flexibility has been explored, where the impact of variability and uncertainty on resource adequacy was outlined and demonstrated using a number of case studies. A specific case study on the impact of flexibility needs on resource adequacy was carried out in 2013 <ref name="F11"></ref>, while 2014 to 2016 work has outlined and performed initial exploration on potential methods <ref name="F13">Flexibility in Transmission and Resource Planning. EPRI, Palo Alto, CA: 2014. 3002003581</ref>, <ref name="F14">System Flexibility Assessment and Analysis. EPRI, Palo Alto, CA: 2015. 3002005763.</ref>, <ref name="F15">Strategic and Flexible System Planning – 2016 Update: Deliverable Flexibility and Flexibility in Resource Adequacy. EPRI, Palo Alto, CA: 2016. 3002008379.</ref>. 2017 work continued investigating the issue of resource adequacy, by expanding on the concept of Installed Flexibility (IFLEX), as described later. In 2018, this concept was further studied using a case study to demonstrate and improve the methods developed, and further investigate how this metric could be used to calculate the system’s ability to meet ramping. |
− | * The impact of the transmission network, and deliverability of flexibility resources, has been researched in more recent years | + | * The impact of the transmission network, and deliverability of flexibility resources, has been researched in more recent years <ref name="F13"></ref>, <ref name="F14"></ref>. The first such study looked at the impact on overall available flexibility when the network was represented in various levels of detail, while more recent work has focused on deliverability of flexibility. At the end of 2018, this is in the process of being added to the Inflexion tool. |
− | * A white paper was released outlining the work EPRI is doing in the area, as well as the flexibility adequacy metrics that were developed, | + | * A white paper was released outlining the work EPRI is doing in the area, as well as the flexibility adequacy metrics that were developed, <ref name="F2">Metrics for Quantifying Flexibility in Power System Planning, EPRI, Palo Alto, CA: 2014. 300200424</ref>. This was part of a larger EPRI-wide initiative on flexibility as a desirable attribute of power systems. |
− | * Throughout this work, case studies have also been carried out to understand these flexibility issues and improve the methods. These include a number of studies in the base research project based on, among others, California, MISO, the Pacific-NW, and supplemental projects including ongoing work with California (PG&E and CAISO), TVA and Eskom and a study with Southwest Power Pool | + | * Throughout this work, case studies have also been carried out to understand these flexibility issues and improve the methods. These include a number of studies in the base research project based on, among others, California, MISO, the Pacific-NW, and supplemental projects including ongoing work with California (PG&E and CAISO), TVA and Eskom and a study with Southwest Power Pool <ref name="F16">Southwest Power Pool, 2016 Wind Integration Study, available https://www.spp.org/Documents/34200/2016%20Wind%20Integration%20Study%20(WIS)%20Final.pdf, 2016</ref>. |
* During case studies, aspects such as the treatment of hydro power and other energy limited resources such as demand response were included, and the functionality of InFLEXion continued to be increased, with new analyses methods added in most years. | * During case studies, aspects such as the treatment of hydro power and other energy limited resources such as demand response were included, and the functionality of InFLEXion continued to be increased, with new analyses methods added in most years. | ||
* Utilities/ISOs have been using InFLEXion themselves in their internal studies, and discussions and interaction with those entities has helped improve the tool, and provided greater understanding of how planners are considering flexibility issues. This includes annual workshops on the topic of flexibility with project members (materials from the workshop are available on the program cockpit on epri.com). | * Utilities/ISOs have been using InFLEXion themselves in their internal studies, and discussions and interaction with those entities has helped improve the tool, and provided greater understanding of how planners are considering flexibility issues. This includes annual workshops on the topic of flexibility with project members (materials from the workshop are available on the program cockpit on epri.com). | ||
Line 74: | Line 74: | ||
EPRI has not been the only entity working in this area. Some of the more relevant industry efforts were described in the past chapter. Additionally, a broad number of different institutions, including national labs, utilities/ISOs, academics, consultants and other industry organizations have worked directly or indirectly in this area. In general, EPRI work has been intended to complement these efforts. Both more detailed and less detailed methods have been proposed and studied. | EPRI has not been the only entity working in this area. Some of the more relevant industry efforts were described in the past chapter. Additionally, a broad number of different institutions, including national labs, utilities/ISOs, academics, consultants and other industry organizations have worked directly or indirectly in this area. In general, EPRI work has been intended to complement these efforts. Both more detailed and less detailed methods have been proposed and studied. | ||
− | In the more detailed cases, production cost model developers and consultants using such tools have been developing methods to explicitly include the need for flexibility in the simulation itself, as opposed to the post processing method EPRI has developed. This has taken the form of including more detail in the inputs, as well as reporting results related to lack of flexibility situations. Examples include the development and use of the REFLEX tool by E3 | + | In the more detailed cases, production cost model developers and consultants using such tools have been developing methods to explicitly include the need for flexibility in the simulation itself, as opposed to the post processing method EPRI has developed. This has taken the form of including more detail in the inputs, as well as reporting results related to lack of flexibility situations. Examples include the development and use of the REFLEX tool by E3 <ref name="F17">J. Hargreaves, E. Hart, R. Jones, A. Olsen, REFLEX: An Adapted Production Simulation Methodology for Flexible Capacity Planning, accessed Nov 2016 at www.ethree.com</ref> and the advancements made to the SERVM tool by Astrape Consulting. More details are given for both in a survey paper that PG&E led and EPRI participated in that was submitted to the California PUC in 2014 <ref>Pacific Gas and Electric, Collaborative Review of Collaborative Review of Planning Models, April 2014.</ref>. |
E3 developed the REFLEX tool to work with existing production cost tools, and has used it with two tools: Energy Exemplar’s PLEXOS and Ecco International’s ProMaxLT software. In both cases, the REFLEX tool was used together with an existing production simulation tool to calculate the need for flexibility under high renewable penetration. REFLEX is used to develop inputs for simulation in the production cost model, and by using Monte Carlo draws of load, wind and solar, can represent the flexibility needs in the simulations. Flexibility deficits can then be identified and examined. | E3 developed the REFLEX tool to work with existing production cost tools, and has used it with two tools: Energy Exemplar’s PLEXOS and Ecco International’s ProMaxLT software. In both cases, the REFLEX tool was used together with an existing production simulation tool to calculate the need for flexibility under high renewable penetration. REFLEX is used to develop inputs for simulation in the production cost model, and by using Monte Carlo draws of load, wind and solar, can represent the flexibility needs in the simulations. Flexibility deficits can then be identified and examined. | ||
Line 82: | Line 82: | ||
While both SERVM and REFLEX are thus somewhat more detailed, in that they explicitly include flexibility needs in the process, they require additional data and new production cost tools. The EPRI method could complement these tools by adding additional metrics to existing software, and it also allows for analysis of real historical data. In the CES-21 project, the InFLEXion tool was used to assess CES-21 results for additional insight beyond typical production cost results of generator production and load/reserve shortfalls. This helped shed light on particular periods of the year and ramping horizons among other aspects, that were tight on flexibility; helping to identify multi-hour ramping needs and additional flexibility metrics. The post processing method also ensures that every hour is examined for worst case events under the conditions assumed, whereas for the tools with flexibility needs integrated, only one actual outcome occurs. The CES-21 project is discussed in more detail in later chapters. | While both SERVM and REFLEX are thus somewhat more detailed, in that they explicitly include flexibility needs in the process, they require additional data and new production cost tools. The EPRI method could complement these tools by adding additional metrics to existing software, and it also allows for analysis of real historical data. In the CES-21 project, the InFLEXion tool was used to assess CES-21 results for additional insight beyond typical production cost results of generator production and load/reserve shortfalls. This helped shed light on particular periods of the year and ramping horizons among other aspects, that were tight on flexibility; helping to identify multi-hour ramping needs and additional flexibility metrics. The post processing method also ensures that every hour is examined for worst case events under the conditions assumed, whereas for the tools with flexibility needs integrated, only one actual outcome occurs. The CES-21 project is discussed in more detail in later chapters. | ||
− | A less detailed approach, though one that still involves simulation of power system operations has recently been developed by the International Renewable Energy Agency (IRENA)<ref>More details on this project and the associated tool can be found at http://irena.org/publications/2018/Nov/Power-system-flexibility-for-the-energy-transition</ref>. They reviewed other approaches, such as those discussed here, and identified a need to develop methods that can answer planner’s questions around flexibility. This includes both looking at investment options and strategies and also looking at an existing or project mix and determining whether it has sufficient flexibility | + | A less detailed approach, though one that still involves simulation of power system operations has recently been developed by the International Renewable Energy Agency (IRENA)<ref>More details on this project and the associated tool can be found at http://irena.org/publications/2018/Nov/Power-system-flexibility-for-the-energy-transition</ref>. They reviewed other approaches, such as those discussed here, and identified a need to develop methods that can answer planner’s questions around flexibility. This includes both looking at investment options and strategies and also looking at an existing or project mix and determining whether it has sufficient flexibility <ref name="F20">A. Mills, J. Seel, Flexibility Inventory for Western Resource Planners, LBNL report, available http://emp.lbl.gov/publications/flexibility-inventory-western</ref>. An open source tool, FlexTool, was developed as part of this project, and has been applied in a number of case studies for various countries. This tool is a linear programming based tool that can be used for investment decisions; it takes a sampling of the full year and attempts to understand ramping and flexibility needs, while also minimizing costs <ref name="F21">IRENA (2018), Power System Flexibility for the Energy Transition, Part 1: Overview for policy makers, International Renewable Energy Agency, Abu Dhabi</ref>. This allows for simpler representation of resources, which could mean the accuracy is not at the same level as some of the previously mentioned tools. However it does allow different investment options, including issues related to energy systems integration (e.g. gas flexibility), to be examined. In operational mode, it behaves a little more like a production cost tool, while still having a simpler underlying algorithm that is designed to provide fast results. It would be expected that this tool could be helpful for policy level analysis, or for initial screening of new flexibility resources that could be examined in more detail using other tools. |
− | Other methods developed in the past few years have also been developed that are simpler than the methods proposed by EPRI, and those used in the production simulation tools described above. These simpler methods range from spreadsheet analysis to methods with some optimization, but with less detail. For example, the International Energy Agency’s Grid Integration of Variable Renewables (GIVAR) project developed both a spreadsheet based tool and one that was based on simulating the flexibility dispatch of the system | + | Other methods developed in the past few years have also been developed that are simpler than the methods proposed by EPRI, and those used in the production simulation tools described above. These simpler methods range from spreadsheet analysis to methods with some optimization, but with less detail. For example, the International Energy Agency’s Grid Integration of Variable Renewables (GIVAR) project developed both a spreadsheet based tool and one that was based on simulating the flexibility dispatch of the system <ref name="F19">International Energy Agency, The Power of Transformation - Wind, Sun and the Economics of Flexible Power Systems. Published 2014, available www.iea.org</ref>. The spreadsheet tool uses input based on the characteristics of the resources on the system, and then attempts to determine the likely ramping capability of the generation fleet. Lawrence Berkley National Lab have taken this concept and developed it further, applying it to the Western US Integrated Resource Plans <ref name="F20"></ref>. The IEA also has developed a simulation method, whereby the generation fleet flexibility is maximized in each hour of the year, to determine how much flexibility is available, with the objective function being to maximize flexibility. |
These IEA methods are similar to the methods proposed by NERC, CAISO, TVA, and others described in the previous chapter, in that it generally aims to assess the flexibility issue by quantitative analysis of historical or simulated data. This is then compared to a relatively subjective measure, typically derived from the premise that as the needs increase, more challenges will be seen. The EPRI flexibility requirements described later are similar in approach (approaches differ in what specific measurements are calculated across all methods, but general concept is the same). Similarly, none of these methods typically addressed detailed operational simulations as is done with EPRI’s AFLEX and DLFEX assessment and the SERVM and REFLEX approaches described above. This is typically due to the fact that in the short term planning horizon, or historical analysis used for many of these does not typically see a large number of very significant challenges that may need to be addressed by new resources. As flexibility needs do increase with increasing renewable penetration, however, more detailed analysis may be needed. This concept of different levels is shown in Figure 2‑5. Methods such as the TVA and Oregon methods are on the left hand side (screening and maximum available ramping), with economic dispatch and generator state methods starting to be considered in NERC, CAISO and IEA methods, and generation characteristics and full studies considered in the detailed IEA, SERVM, REFLEX and detailed EPRI methods. | These IEA methods are similar to the methods proposed by NERC, CAISO, TVA, and others described in the previous chapter, in that it generally aims to assess the flexibility issue by quantitative analysis of historical or simulated data. This is then compared to a relatively subjective measure, typically derived from the premise that as the needs increase, more challenges will be seen. The EPRI flexibility requirements described later are similar in approach (approaches differ in what specific measurements are calculated across all methods, but general concept is the same). Similarly, none of these methods typically addressed detailed operational simulations as is done with EPRI’s AFLEX and DLFEX assessment and the SERVM and REFLEX approaches described above. This is typically due to the fact that in the short term planning horizon, or historical analysis used for many of these does not typically see a large number of very significant challenges that may need to be addressed by new resources. As flexibility needs do increase with increasing renewable penetration, however, more detailed analysis may be needed. This concept of different levels is shown in Figure 2‑5. Methods such as the TVA and Oregon methods are on the left hand side (screening and maximum available ramping), with economic dispatch and generator state methods starting to be considered in NERC, CAISO and IEA methods, and generation characteristics and full studies considered in the detailed IEA, SERVM, REFLEX and detailed EPRI methods. | ||
Line 170: | Line 170: | ||
As described, one can end up with a number of different means to determine flexibility requirements. For example, for large changes in net load, it may be more straightforward to determine the overall net load variability, by time of day or year. This can be compared to available flexibility, as described later, to determine whether flexibility is sufficient. On the other hand, using specific wind, solar and load characteristics can give a more accurate representation of what the specific needs at a given time interval may be, particularly when examining these issues in operations or operational simulations. These can then be combined, assuming no correlation, using a geometric sum, which is the square root of sum of squares (it is not recommended to just add wind, solar and load together directly as this would overestimate requirements). The InFLEXion tool currently has capability to determine ramping requirements for each individual source (wind, solar, load) separately, or as a combined net load variability. Both are recommended to be examined, with the user determining flexibility needs based on individual components or combined net load based on their specific results and system. | As described, one can end up with a number of different means to determine flexibility requirements. For example, for large changes in net load, it may be more straightforward to determine the overall net load variability, by time of day or year. This can be compared to available flexibility, as described later, to determine whether flexibility is sufficient. On the other hand, using specific wind, solar and load characteristics can give a more accurate representation of what the specific needs at a given time interval may be, particularly when examining these issues in operations or operational simulations. These can then be combined, assuming no correlation, using a geometric sum, which is the square root of sum of squares (it is not recommended to just add wind, solar and load together directly as this would overestimate requirements). The InFLEXion tool currently has capability to determine ramping requirements for each individual source (wind, solar, load) separately, or as a combined net load variability. Both are recommended to be examined, with the user determining flexibility needs based on individual components or combined net load based on their specific results and system. | ||
+ | |||
+ | == References and Footnotes == |
Latest revision as of 09:30, 15 September 2020
The need for flexibility is impacting both planning and operational processes. In operations, this manifests itself in areas such as operating reserves, and scheduling practices that consider flexible resources. For example, new reserve products have been proposed in many regions to procure ramp capability in the real time and day ahead markets. In this document, the focus is more on how the need for flexibility interacts with planning processes that decide when to build or procure new resources, and/or develop new incentives such as a market product. Some of the considerations in that interaction relate to how those resources will be operated, and so one of the key considerations is how much operational detail needs to be included in planning models. The chapter highlights the areas of interaction between flexibility and existing planning functions and then summarizes work to date by EPRI and its members in the area, and puts this in the context of other ongoing efforts across the industry.
Contents
- 1 How planning functions are being impacted
- 2 Integrated Resource Planning (IRP) and generation expansion methods
- 3 Resource Adequacy Studies
- 4 Need to reflect operational detail in planning processes
- 5 Summary of previous EPRI work
- 6 Context of EPRI tools versus other approaches
- 7 Guide to: Assessing and Screening Flexibility Requirements
- 8 References and Footnotes
How planning functions are being impacted
A number of traditional planning functions and entities are being impacted by the need for flexibility, as shown in Figure 2‑1. In some cases, this is leading to minor adjustment of processes to better represent flexibility, while in other cases, particularly those where wind and solar penetrations are expected to hit relatively high levels (e.g. over 20% energy penetration in next 10 years), new processes have been proposed, or existing processes have been redesigned.
Integrated Resource Planning (IRP) and generation expansion methods
This refers to the planning processes used to determine the future plant mix on the system. Starting with a projected energy and peak power demand, load profile, the current system and potential expansion options, these studies strive to understand the need for new resources. New resources can be built to meet peak demand, while considering uncertainty in demand growth, fuel prices, generation retirement and generation outages, or resources could be built to more efficiently meet load energy demand in future years using less expensive technologies. The need for flexibility has always been inherent in this process, but generally represented by ensuring a suitable mix of baseload, mid merit and peaking resources. In recent years, policy outcomes are becoming more important, while coal retirement has become a major issue. The deregulation of the power system has also impacted how these are carried out, with more Independent Power Producers, and the need to coordinate plans between a utility, the ISO (if present) and state regulators.
Flexibility needs have the potential to impact IRP and other generation expansion studies more than any other area. With increasing variability and uncertainty, it may no longer be sufficient to build out resources in the same manner. Peak demand may become less of an issue with wind and solar being energy and not capacity type resources. At the same time, understanding whether the system has sufficient ability to move resources to respond to increasing ramping needs is important. The increased flexible operations needed, as well as the need to deal with uncertainty in net demand, will result in more value being placed on flexible resources. This could come in the form of a constraint whereby a certain amount of flexibility is required; however that raises the question of how one defines flexibility needs and resources, as discussed later. It may also impact the economics of different resource types, where previously uneconomic resources such as energy storage may become more valuable, and inflexible base load units less valuable. The pace at which this change happens will be specific to a given area and its existing resources, and so resource planners will need to be able to represent their system with sufficient detail to represent the value of flexibility.
Resource Adequacy Studies
Resource Adequacy studies, while strongly related to resource planning, are more focused on the reliability aspects of planning. Here, the ability of resources in a given area (typically balancing authority, but states, interconnections, etc., could also be examined) to meet peak demand is calculated. This can be done either for the existing system, with some load growth, or the expected future system, with additions and/or retirements considered. Two main methods are used to perform detailed studies i) detailed chronological modeling, where the operation of the system is studied for each hour or suitable interval of the year; and ii) snapshot modeling where the likelihood of being able to meet peak demand is calculated. Probabilistic methods are commonly used to include the likelihood of outages of different resources, and typical metrics are Loss of Load Expectation (LOLE) or Expected Unserved Energy (EUE). The capacity credit of resources can be calculated by determining how the resource is expected to contribute to peak demand.
With the increased wind and solar penetration, these methods become more complex. The ability of any one wind and solar plant to meet peak demand is not independent from the other wind and solar plants, and both may also be related to each other and to load due to underlying weather patterns. So determining the likelihood of meeting peak demand becomes more data-intensive process. Methods have been outlined in the literature and are in use in many areas to calculate the capacity credit of wind and solar plants.
Even beyond this issue, however, wind and solar may also impact on resource adequacy studies as their variability and uncertainty may cause periods when, even though sufficient capacity may be available, there is insufficient flexibility. This provides the motivation for much of the studies in this guidelines document, where flexibility sufficiency needs to be assessed to ensure that situations do not occur more often than desired where, even though installed capacity is sufficient, ramping capability is lacking. As described before, this then requires more detailed consideration of system operations in the resource planning timeframe.
As an example of this additional detail that is required, in 2013 EPRI studied the issue of resource adequacy with high levels of renewables using the SERVM tool, as run by Astrape Consulting [1]. This is a probabilistic production cost tool, using thousands of annual production cost runs to identify loss of load expectation (LOLE) and similar metrics. For the 2013 study, the short term uncertainty (day ahead, hour ahead, etc.) associated with wind and solar power was included in the model, and the impact of this on the loss of load was determined. This was done for a high solar and wind penetration case in California. As shown in Figure 2‑2, this resulted in additional loss of load compared to a case where renewables were assumed to be forecasted perfectly. This shows that if uncertainty is not considered in the model, the LOLE may be underestimated by 0.75 days per year (in that particular study), which could result in a system not able to meet the required standards, or that may require additional capacity.
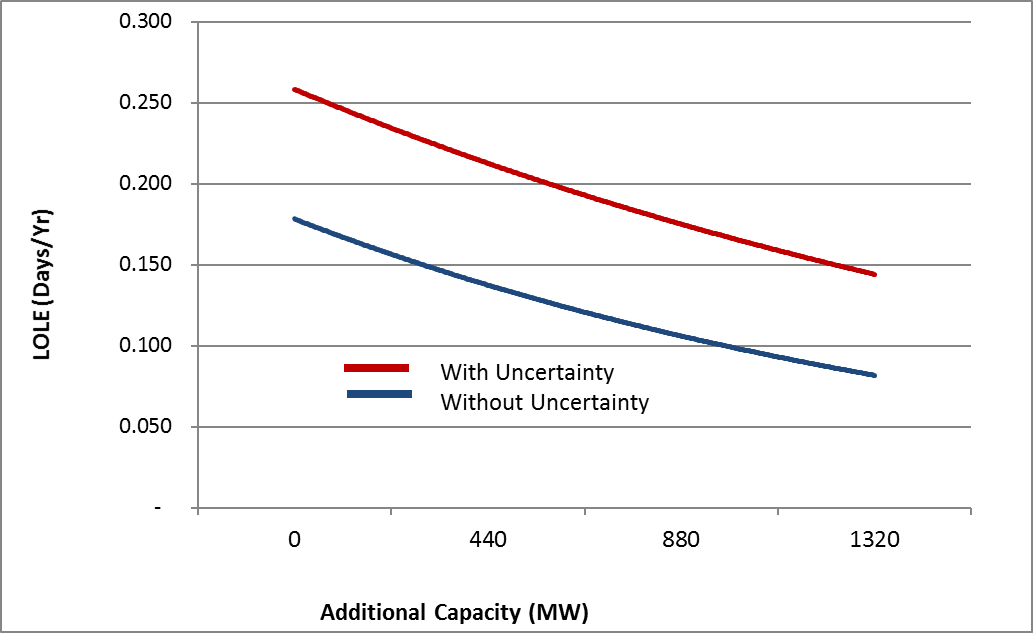
While it is not clear how this should impact on planning standards (as discussed later in the summary of the CES-21 project), and whether the same LOLE standard should be used to ensure sufficient flexibility as used for capacity (i.e. whether one would want to still maintain a loss of load of 1 day in ten years, even when adding consideration of ramping into the calculation), it is nonetheless clear that the variability and uncertainty of renewables has the potential to impact on resource adequacy, leading to increased need to consider these issues. The SERVM tool is discussed in numerous places later, in particular for discussion of the CES-21 project in California, where it was used to investigate the need for flexibility metrics to assist planning processes.
Transmission Planning and Deliverability
Transmission Planning activities typically look at the need for new transmission resources, and interact with resource planning and resource adequacy. Knowing where resources in the future are going to be located allows assessing if the existing and future transmission system is able to reliability and efficiently deliver energy. This is done using both power flow/stability and production cost tools. Projects can be used to improve reliability (i.e. to manage contingencies on the system), improve economics (i.e. reduce congestion and improve dispatch), or a combination of both. Transmission planning studies are typically carried out by ISOs or vertically integrated utilities, often interacting with transmission owners (merchant and regulated). Newly proposed projects have to show a sufficiently positive cost-benefit ratio in order to be approved.
With increasing levels of wind and solar power, the needs for transmission can be significantly impacted. The location of the resources is now typically further from load (or on the distribution system), and so power flows are altered significantly. With increasing need for flexibility, the need to assess whether and how the system can access that flexibility will become increasingly relevant. Additionally, there may be increased value in building network infrastructure between regions to allow for greater coordination to reduce the impacts of variability and uncertainty. As such, the topic of flexibility may become more important for transmission planning processes. While there has always been a strong link between production cost modeling and transmission planning, the need to ensure system operations are adequately represented will increase, and transmission planners will need methods to be able to value the flexibility of their system to integrate renewables. EPRI has recently developed methods to consider the deliverability of flexibility, as discussed in later parts of this report.
Policy and other analyses
Policy analysis typically aims to understand how different policy options can impact on the system, and are carried out by government organizations or similar bodies to look at higher level questions. Traditionally, this has been done in the form of higher level tools that model the impact of the electricity network on the broader society, such as EPRI’s US-REGEN tool. Different load growth assumptions, costs of technologies and policy preferences are modeled at a high level and overall plant mixes are determined. With increasing variability and uncertainty, such analyses may need to consider whether the flexibility in the fleet is sufficient. Unlike more detailed resource planning studies, specific information about the operations of individual plants, and the need for specific new flexibility may not be as important here as a general conclusion about the overall changes required to manage the variability and uncertainty. Therefore, when looking at this type of time horizon (15-20 years plus), it may be sufficient to use screening techniques mixed with existing methods. However, may still want to consider whether the system is shown to change sufficiently that more detailed study is warranted.
Need to reflect operational detail in planning processes
As described above, different parts of the planning process are being impacted by issues related to operational flexibility. In general, planning for areas with high levels of renewables expected to come online will need to be adopted. Conceptually, Figure 2‑3 represents a potential means to consider flexibility in a more detailed manner in the planning process.
Generally, it can be seen that this is done by analyzing flexibility as an additional consideration after considering more typical generation and transmission planning processes. It may be that, in time, flexibility may be subsumed into the existing areas, such that, installed flexibility requirements are automatically a part of the capacity adequacy process. It may also be that in some regions, the flow chart may not always follow this exact process, for example, installed flexibility may be considered before network adequacy. As can be seen in the figure, flexibility could eventually be considered as an additional analysis in all aspects of generation (and other resources such as demand response or energy storage) and transmission planning. It may be that it is not always considered in as much detail as shown here, depending on the expected impact that flexibility needs may have on the planning processes. Different flexibility analyses on this chart correspond to the various flexibility assessment described later.
Note that in some areas, resource and transmission expansion may be iterative, and in future may even move to being done as a co-optimization, with non-transmission resources also being considered at the same time as transmission. The figure shows both existing planning processes and tools as well as those new methods and tools that are needed to ensure sufficient operational flexibility, identified by either an existing or future function in the InFLEXion planning tool.
Moving through the flowchart, the first two steps are based on a typical planning process, whereby a long-range future is determined (this is mainly load forecast, but may include policies and other factors). Then, resource adequacy is assessed and additional generation needs identified. Based on their location, transmission needs are then assessed. Then, the installed flexibility (IFLEX) is calculated, as described in Chapter 5, to determine whether (regardless of economics) additional flexible resource buildout is needed to meet desired levels of reliability. If sufficient flexibility is available without consideration for how the system would really be operated, then the next step may be to use a detailed production cost model of system operations to further identify the available flexibility (AFLEX) that would be expected based on assumed operational practices. The use of production cost modeling is described in Chapter 4. Results from this step may inform both resource and transmission buildout, where it may be economic to add flexibility resources based on simulation results. This step may also help identify scheduling design practice changes to accommodate the need for increased flexibility. Finally, the deliverability of the flexibility (DFLEX) is assessed, by considering how the network impacts on delivery of flexibility.
Not everyone would use this chart the same way, similar to how not everyone will plan in the same way. For example, in an energy only electricity market, as there is no means to procure capacity directly, these studies may be more useful to identify needs and likely shortfalls, to examine the transmission network, and to determine how prices may evolve. Similarly, vertically integrated utilities may need to build out resources and transmission at lowest cost while maintaining reliability, whereas this approach may be used by Independent Power Producers to identify resources that could be profitable in the future. Transmission planners may use these methods to better understand their needs, while resource adequacy studies may be more focused on the generation resource parts of the figure. This conceptual figure is intended to provide an overall look at how one should think about issues relating to flexibility. It is clear from this figure how, for systems with increasing levels of wind and solar power, this topic could involve significant changes and additional iterations to their planning processes.
One of the most important short-term changes to make will be to more accurately consider operations in the planning time frame. As wind and solar power are both variable and uncertain, there is a need to model this in sufficient detail in order to understand where the flexibility to manage this will come from. As time goes by and planners become more confident in their methods, they may be able to return to using rules of thumb or other simple practices, but it is likely that, as the transition to a higher renewable generation mix is underway, methods will need to become more advanced.
Summary of previous EPRI work
For the past several years, EPRI has performed work in the project, “Strategic and Flexible Planning” on the topic of operational flexibility. This was part of the Grid Planning (P40) program from 2011 until 2014, and from 2015 moved to be part of the Bulk System Integration of Variable Generation (P173) program. The focus has been on understanding the need for and provision of operational flexibility in power system planning, focusing on a number of aspects, as summarized in Figure 2‑4:
- Initial work used case studies to illustrate the need for flexibility and develop some initial concepts and a framework for assessment of the flexibility on the system [2][3][1]. This included analyzing results from production cost tools to better understand flexibility issues, and introduced concepts such as consideration of different time horizons, use of different percentiles in assessing flexibility requirements, and the need to examine both upwards and downwards ramping.
- Based on these initial studies, metrics and tools were developed to assess the needs for and provision of flexibility. This led to the creation of the InFLEXion tool, which has continued to improve over numerous versions. The latest version of InFLEXion, v5.1, is expected to be released in late 2018. It is likely that a user’s group will also be started in next few years.
- Resource adequacy and its links with flexibility has been explored, where the impact of variability and uncertainty on resource adequacy was outlined and demonstrated using a number of case studies. A specific case study on the impact of flexibility needs on resource adequacy was carried out in 2013 [1], while 2014 to 2016 work has outlined and performed initial exploration on potential methods [4], [5], [6]. 2017 work continued investigating the issue of resource adequacy, by expanding on the concept of Installed Flexibility (IFLEX), as described later. In 2018, this concept was further studied using a case study to demonstrate and improve the methods developed, and further investigate how this metric could be used to calculate the system’s ability to meet ramping.
- The impact of the transmission network, and deliverability of flexibility resources, has been researched in more recent years [4], [5]. The first such study looked at the impact on overall available flexibility when the network was represented in various levels of detail, while more recent work has focused on deliverability of flexibility. At the end of 2018, this is in the process of being added to the Inflexion tool.
- A white paper was released outlining the work EPRI is doing in the area, as well as the flexibility adequacy metrics that were developed, [7]. This was part of a larger EPRI-wide initiative on flexibility as a desirable attribute of power systems.
- Throughout this work, case studies have also been carried out to understand these flexibility issues and improve the methods. These include a number of studies in the base research project based on, among others, California, MISO, the Pacific-NW, and supplemental projects including ongoing work with California (PG&E and CAISO), TVA and Eskom and a study with Southwest Power Pool [8].
- During case studies, aspects such as the treatment of hydro power and other energy limited resources such as demand response were included, and the functionality of InFLEXion continued to be increased, with new analyses methods added in most years.
- Utilities/ISOs have been using InFLEXion themselves in their internal studies, and discussions and interaction with those entities has helped improve the tool, and provided greater understanding of how planners are considering flexibility issues. This includes annual workshops on the topic of flexibility with project members (materials from the workshop are available on the program cockpit on epri.com).
- EPRI has participated in a number of efforts related to flexibility assessment. This includes work done by the Northwest Power and Conservation Council (NWPCC) on consideration of flexibility in the resource plans for the northwest, as well as contributions to various studies and papers by NREL, Ecofys, LBNL, and NERC.
Context of EPRI tools versus other approaches
EPRI has not been the only entity working in this area. Some of the more relevant industry efforts were described in the past chapter. Additionally, a broad number of different institutions, including national labs, utilities/ISOs, academics, consultants and other industry organizations have worked directly or indirectly in this area. In general, EPRI work has been intended to complement these efforts. Both more detailed and less detailed methods have been proposed and studied.
In the more detailed cases, production cost model developers and consultants using such tools have been developing methods to explicitly include the need for flexibility in the simulation itself, as opposed to the post processing method EPRI has developed. This has taken the form of including more detail in the inputs, as well as reporting results related to lack of flexibility situations. Examples include the development and use of the REFLEX tool by E3 [9] and the advancements made to the SERVM tool by Astrape Consulting. More details are given for both in a survey paper that PG&E led and EPRI participated in that was submitted to the California PUC in 2014 [10].
E3 developed the REFLEX tool to work with existing production cost tools, and has used it with two tools: Energy Exemplar’s PLEXOS and Ecco International’s ProMaxLT software. In both cases, the REFLEX tool was used together with an existing production simulation tool to calculate the need for flexibility under high renewable penetration. REFLEX is used to develop inputs for simulation in the production cost model, and by using Monte Carlo draws of load, wind and solar, can represent the flexibility needs in the simulations. Flexibility deficits can then be identified and examined.
The SERVM tool, a probabilistic production cost tool that has traditionally been used for reliability analysis and resource adequacy studies, has had several features added in recent years to improve representation of flexibility needs. Most relevant, it was recently used in a project under the California Energy Systems for the 21st Century (CES-21) program, on flexibility metrics and assessment. That project aimed to identify whether California’s resource planning and procurement processes need to include specific flexibility metrics, and identify potential approaches for this. As such, SERVM was used to test various approaches and potential means to assess flexibility. This includes detailed representation of intra- and inter-hour variability and uncertainty. By examining these issues, the likelihood of flexibility deficits can also be seen in the results of the probabilistic production cost simulations. SERVM typically analyzes thousands of cases (for different outage patterns, wind/solar load patterns, load growth, etc.), and thus can provide useful stochastic results on the issues relating to flexibility.
While both SERVM and REFLEX are thus somewhat more detailed, in that they explicitly include flexibility needs in the process, they require additional data and new production cost tools. The EPRI method could complement these tools by adding additional metrics to existing software, and it also allows for analysis of real historical data. In the CES-21 project, the InFLEXion tool was used to assess CES-21 results for additional insight beyond typical production cost results of generator production and load/reserve shortfalls. This helped shed light on particular periods of the year and ramping horizons among other aspects, that were tight on flexibility; helping to identify multi-hour ramping needs and additional flexibility metrics. The post processing method also ensures that every hour is examined for worst case events under the conditions assumed, whereas for the tools with flexibility needs integrated, only one actual outcome occurs. The CES-21 project is discussed in more detail in later chapters.
A less detailed approach, though one that still involves simulation of power system operations has recently been developed by the International Renewable Energy Agency (IRENA)[11]. They reviewed other approaches, such as those discussed here, and identified a need to develop methods that can answer planner’s questions around flexibility. This includes both looking at investment options and strategies and also looking at an existing or project mix and determining whether it has sufficient flexibility [12]. An open source tool, FlexTool, was developed as part of this project, and has been applied in a number of case studies for various countries. This tool is a linear programming based tool that can be used for investment decisions; it takes a sampling of the full year and attempts to understand ramping and flexibility needs, while also minimizing costs [13]. This allows for simpler representation of resources, which could mean the accuracy is not at the same level as some of the previously mentioned tools. However it does allow different investment options, including issues related to energy systems integration (e.g. gas flexibility), to be examined. In operational mode, it behaves a little more like a production cost tool, while still having a simpler underlying algorithm that is designed to provide fast results. It would be expected that this tool could be helpful for policy level analysis, or for initial screening of new flexibility resources that could be examined in more detail using other tools.
Other methods developed in the past few years have also been developed that are simpler than the methods proposed by EPRI, and those used in the production simulation tools described above. These simpler methods range from spreadsheet analysis to methods with some optimization, but with less detail. For example, the International Energy Agency’s Grid Integration of Variable Renewables (GIVAR) project developed both a spreadsheet based tool and one that was based on simulating the flexibility dispatch of the system [14]. The spreadsheet tool uses input based on the characteristics of the resources on the system, and then attempts to determine the likely ramping capability of the generation fleet. Lawrence Berkley National Lab have taken this concept and developed it further, applying it to the Western US Integrated Resource Plans [12]. The IEA also has developed a simulation method, whereby the generation fleet flexibility is maximized in each hour of the year, to determine how much flexibility is available, with the objective function being to maximize flexibility.
These IEA methods are similar to the methods proposed by NERC, CAISO, TVA, and others described in the previous chapter, in that it generally aims to assess the flexibility issue by quantitative analysis of historical or simulated data. This is then compared to a relatively subjective measure, typically derived from the premise that as the needs increase, more challenges will be seen. The EPRI flexibility requirements described later are similar in approach (approaches differ in what specific measurements are calculated across all methods, but general concept is the same). Similarly, none of these methods typically addressed detailed operational simulations as is done with EPRI’s AFLEX and DLFEX assessment and the SERVM and REFLEX approaches described above. This is typically due to the fact that in the short term planning horizon, or historical analysis used for many of these does not typically see a large number of very significant challenges that may need to be addressed by new resources. As flexibility needs do increase with increasing renewable penetration, however, more detailed analysis may be needed. This concept of different levels is shown in Figure 2‑5. Methods such as the TVA and Oregon methods are on the left hand side (screening and maximum available ramping), with economic dispatch and generator state methods starting to be considered in NERC, CAISO and IEA methods, and generation characteristics and full studies considered in the detailed IEA, SERVM, REFLEX and detailed EPRI methods.
An initial attempt to lay these out is shown in Figure 2‑6, with level of operational detail compared to type of analysis. While one would ideally be on the top right, it is important to be able to perform other studies as appropriate, based on time and data available, and importance of the flexibility issue.
Guide to: Assessing and Screening Flexibility Requirements
The purpose of assessing flexibility requirements for the system under study is to inform detailed studies, operational processes or to screen for flexibility sufficiency. This corresponds to Level 1 analysis in the InFLEXion tool. Here, flexibility requirements primarily refer to net load ramping capability needed by the system, which may increase with wind and solar penetration. In some cases, it may also be instructive to look at wind, solar and load ramping separately.
A major reason one would need to assess flexibility requirements in a planning context would be as an input to detailed planning tools, such as production cost models described in later chapters. By carefully determining the net load ramping requirements that are associated with future system mixes, one can then determine if there is sufficient flexibility in the system to meet the requirements. Flexibility requirements will often show up in such studies as reserve requirements, which could be in the form of the magnitude of existing reserves such as frequency regulation or spinning/non-spinning, or could take the form of new reserve types. For example, load following reserves are used in many studies, covering a variety of time horizons or interval lengths, and methods described here could inform such requirements. At the same time, those studies may also identify if flexibility is short and can help to determine if there are additional requirements for reserves or for investment in new capacity.
As well as input to production cost modeling, flexibility requirements may also be useful to assess for screening methods such as the NERC and IEA approaches described earlier. By carefully determining assumptions used in calculating these requirements, as described in this section, these screening methods can tell a lot about needs now and in the future. For example, the methods described below were applied to two Southeastern US footprints (TVA and Southern Company) as part of a recent DOE-funded study looking at future solar penetrations. While further detailed analysis was not completed as part of that project, analysis of requirements provided a first step in understanding ramping needs and the type of behavior that may be expected as solar penetration increases, and provided useful information for planners there to understand how solar may impact on their flexibility needs.
The final reason to understand flexibility requirements is for post processing of historical or simulated data, as described in later chapters. Here, the requirements identified can be compared to what is actually provided by the system dispatch, to ensure flexibility is available. By analyzing trends into the future, one can determine how ramping needs are changing over time, and whether the system will require additional flexibility.
Recommendations for assessing flexibility requirements
Flexibility requirements, whether used to determine reserve needs in production cost studies, as an input to screening methods, or for post processing studies to determine flexibility sufficiency, should consider a number of factors, as described here. Typically, these are based on net load ramping, which is the system demand minus the variability and uncertainty caused by wind and solar, and potentially could also include other factors such as fixed interchange schedules.
Time horizons studied
As shown with the various examples earlier, a number of time horizons have been proposed to study flexibility issues. It is recommended to study multiple time horizons in order to identify different flexibility issues. This typically includes anything from 5-minutes (or even 1-minute) to several hours. The main focus should be on understanding operational issues, particularly related to economic dispatch. Thus, one should ideally study the dispatch interval as the shortest time horizon. As one considers flexibility needs, longer time horizons than the dispatch interval are also important. This is to ensure commitment and dispatch processes have sufficient flexibility to meet forecasted and unexpected changes in net load. While systems differ as to what intervals should be studied, the recommended time horizons typically include:
- 5- or 10-minutes: is typically when wind and solar start to have an impact on overall system ramps. Within this interval, regulating reserves can be used to manage variability.
- 20- or 30-minutes: This is a typical load following time horizon, where dispatch will need to consider expected changes. Fast start resources can also be committed in this time horizon. Note others may call this a ‘flexibility’ reserve and load following may refer to longer time horizons, and dispatch of resources is also an important factor here.
- 1-hour: This is a typical resolution at which operators determine day-ahead (or days ahead) operating plans. Many datasets may not be higher time resolution than this, and often payments and metering is based on this time interval.
- 3- or 4-hour: This time horizon is a typical start up time for combined cycle gas generation, and is also a period during which forecasting of wind and solar may become more accurate compared to day ahead. This is often the last chance to make decisions about committing larger units to manage forecast error. Additionally, this time horizon will cover issues such as the ‘duck curve’ seen in California, where solar output decreases at the end of the day, and is typical of the length for the largest up and down ramps in net load.
- 6- to 8-hours: This longer time horizon is useful for a number of reasons. On the one hand, longer starting generators can take this long to start up, e.g. in the Irish system, Eirgrid is now incentivizing 8-hour ramping capability due to a number of units that start in 7 to 8 hours. On the other hand, this time horizon is typical in many systems of the difference in time between high and low load in the day. For example, this is the difference between load in the middle of the night and early morning, or between peak demand in late afternoon and late at night/early morning. As such, one should choose a time period that reflects both physical capability and changes in net load over longer durations.
As well as above horizons, others may also be important for a given system. For example, the day ahead flexibility issues may also require a 24-hour assessment of uncertainty needs, but not focus as much as variability, in order to understand typical forecast errors. In general, a recommended practice would be to study all time horizons of interest first, and then focus on those of most relevant for a given system. The InFLEXion tool can be used to study all time horizons, and typically once one completes a number of studies, one can determine a suitable set of horizons as described below and later in the section on interpreting results.
An example of the analysis of different time horizons is shown in Figure 3‑1. Here, only one-hour data was available, so that was used as shortest horizon. Looking at results, it can be seen that the largest ramps in all types of data (variable generation, which in this case was wind, load and net load) maintain an almost constant rate of increase as a function of the horizon up to 8 h. There is a drop off in the rate of increase as horizon gets larger (after 8 h). For example, the largest net load ramps (shown in green) get 5 GW larger when going from 2 hours to 4 hours, but only about 8 GW larger when moving from 4 hours to 6 hours. Here, time horizons of interest could be suggested as 1 hour, 4 hours and 7 or 8 hours. At around 8 hours, the net load up ramps see a significant increase compared to load, so that would be of interest. One should also consider system capabilities in setting the time horizons.
Percentile approach to flexibility requirements
One of the key concepts in assessing the variability and uncertainty of load, wind/solar output or net load is that of percentiles. The concept is that one would like to cover a certain amount of the variability and/or uncertainty that would be expected in the future. By examining historical or simulated data, one can determine percentiles of data. For example, the 99th percentile is the value observed which is larger in magnitude than 99% of all other data. This can be used to assess either variability or uncertainty.
Typically, studies try to link the percentiles with system reliability criteria. For example, if the Area Control Error (ACE) is only allowed outside a certain band 5% of the time over a given period, one may want to use a 95th percentile of requirements. However, it should be noted that, even were one to use this requirement these two things are not equivalent, as in actual operations other resources may be available, and as such detailed operational simulations are still required. However, percentiles give a first approximation at what may be studied. As such, percentiles should be varied when performing screening analysis, typically, 95th and 99th, or even 99.9th or 99.99th are used to provide bookends.
Longer duration ramps may be addressable in operations by adjusting dispatch and commitment decisions, and forecasts can also be used, to ensure that operators are aware a large ramp may occur and rearrange the dispatch of resources as necessary. Thus it may be appropriate there to not use as high a percentile if only assessing variability; on the other hand, when it comes to very short term decisions, or when uncertainty is being assessed, higher percentiles may make more sense. Additionally, whether neighboring regions can be leaned on to a certain extent, or whether there is some interruptible load or other demand side resources may mean that requirements can be relaxed somewhat. An isolated system that strives not to shed even a small amount of load will need tighter requirements than a less constrained area.
Figure 3‑2 shows an example of the use of percentiles for solar variability. The first thing to notice is how much larger the largest ramp is compared to the 99th percentile, in both up and down directions, in one minute horizons. This is due to the fact that, in the data used, there was a large change in a very small number of hours. This data was simulated based on actual solar irradiance, and shows that, if one seeks to cover all one minute ramps in every hour, the ramp requirements may be significantly larger than would be needed during the vast majority of hours of the year. On the 60-minute horizon, and to a lesser extent the 10-minute horizon, this effect is not as apparent, but the changes for different percentiles are still quite different.
Another useful use of percentile requirements, based on this effect, to understand how resources may be used. For example, if 95th and 99th percentile of annual hourly data showed similar numbers, that would imply that the 88th largest ramp (8760 divided by 100) are not too much larger than the 440th largest ramp. As such, resources sized to cover these ramps may need to be available more frequently, and the cost of dispatching them is more important. If 99th percentile ramp was significantly higher, then maybe a less economic resource in terms of dispatch cost, but with low capital cost, could be used (e.g. demand response product or diesel engines).
This may imply that if a study was more focused on reliability, then the percentile used should be based on reliability measures (either long term planning such as 1-day-in-10 or operational methods such as CPS scores) . Studies focused on economic resource expansion should look at the frequency of shortfall before determining needs. For example, one could assess 90th percentile and use that to determine requirements for mid-merit generation, but use a 99th percentile to determine demand response needs; in a market context, one may set penalties differently based on the percentile, similar to a demand curve for shortages. As noted in the concluding chapter, the methods for resource adequacy and resource expansion to consider flexibility are still evolving, so this use of percentiles is still to be further development.
Use of conditional requirements
When actually determining the ability of the system to procure sufficient flexibility, it will be important to recognize that the flexibility requirements should vary. For example, if one were to use the 99th percentile of all ramps over a given time horizon in all hours of a study, then there are likely some hours when this would be overestimating flexibility needs; an example is where solar is considered, there is no need to require large amounts of reserves to cover solar variability and uncertainty in the nighttime hours. Similarly, there may be periods when wind is producing at very low levels, such that the need to cover down ramps in wind (up ramps in load) is unnecessary.
Time of Day/Year
The flexibility requirements may vary based on time of day or time of year. For example, different seasons may see different requirements for horizons and direction of ramping. In order to determine requirements in this fashion, there is a trade-off between granularity and the amount of data available. For example, seasonal requirements are often proposed, as they provide a good mix between significant amounts of data available if looking over a few years, and a relatively granular approach. Seasons may not directly line up with the four seasons typically considered, but may be based on different regimes across the year. For instance, one may want to consider December and January alone in some regions, or may include November and February in others; the main point is to group similar months. Since weather from one month to another within a season should be relatively consistent, it may make sense to use seasonal rather than monthly requirements. At the same time, there may be monthly variations that do need to be captured.
Figure 3‑3 shows an example of a result from previous year’s work, where net load variability was studied by time of day and month of year. As shown, there seems to be a pattern as to when the largest ramps happen. Winter and spring mornings undergo large ramping, while the afternoon is more impacted in the summer. This is likely due in this specific case to a particular combination of the load profile and the wind penetration and pattern assumed, and certainly would not be true in other areas. However, such a result could be used to determine when flexibility may be required.
It is thus recommended to study requirements by both month and season (and to adapt specific definitions for ‘season’). Examining how these requirements vary should allow insight into the better method to use. For example, if requirements vary significantly across months, but no underlying reason can be determined (e.g. July and August are very similar, but September is far greater), then it may be that the relative lack of data is causing some outliers, rather than showing a real variation in requirements. On the other hand, if the monthly requirements seem relatively consistent (e.g. August is close to, but only a little higher than July and September), then monthly requirements may give more detail and allow for more efficient determination of needs. Typically, having more data (e.g. decades of wind/solar/load) should allow for monthly data, whereas less than two or three years may require a seasonal approach.
Production or Load Level
Another manner that can be used to determine flexibility requirements, and could be combined with time of day/year-type requirements, is to base the requirements on load, wind, solar or net load level. This concept has been developed in integration studies and can be used to manage variability, uncertainty, or both. The main idea is to examine historical data (variability and/or uncertainty), and then determine relationships between flexibility requirements and the condition at the start of the time horizon.
The main concept for condition based requirements is to determine the relevant explanatory variables upon which one can base flexibility requirements. For example, wind power, based on its power-speed curve, tends to be most variable when the output is close to half of its capacity at the start of a given horizon. Thus the main variable should be normalized wind power output. Load variability tends to be more based on time of day, but can also depend on load level; one potential approach could be to split data up into time of day and then load level.
Figure 3‑4 shows an example based on a previous EPRI study on wind power variability. This is based on covering the 99th percentile of flexibility in an historical dataset. This dataset was first binned into 10 different production levels (0%-10%, 10%-20%, etc.) based on production at the start of the hour. Then, data within each level was examined for the 99th percentile of ramp magnitude. The figure shows that, except for very long ramps, the requirements for both up and down ramping tend to be greatest when wind output is approximately 50% of capacity. For 4 hour ramps, the largest net load up ramps happen when wind output is high, whereas for lower wind output levels, the system needs to be able to ramp down. What this shows is that, for this dataset, it takes longer than a few hours to see wind ramp from a high output to close to zero, and vice versa. On an hourly or lower horizon, large variability is driven more by the variation in the middle of the power-speed curve.
Solar PV has a number of potential explanatory variables. While output level could be used similar to wind, the underlying shape of solar can also allow for greater understanding of needs. This uses the concept of “Clear Sky Output”, which is what the expected output would be (neglecting temperature impacts) were the PV panels producing at maximum output, with no clouds. This underlying shape can then be examined to determine “Solar Power Index (SPI)”, the ratio of actual output to Clear Sky, as shown in Figure 3 5. Data can then be binned, similar to the wind data. However, rather than binning for just one variable as with wind, it would be binned based on two variables. If each variable had 10 different levels, that would mean 10×10 = 100 bins of data. Thus selecting bins so that there is a sufficient amount of data in each would be important; for example, one may want to divide SPI into 10 bins, but clear sky ramp into only 5, to give 50 bins. This data can then be analyzed to determine, for a given percentile, what up and down ramping required would be in each bin. As SPI and clear sky ramp account for the underlying solar shape, this means only the deviations from this shape contribute to requirements. This can be useful in determining flexibility requirements to manage these unexpected (though partly forecastable) variations. However, one may still want to also examine the absolute changes in solar, as these will still need to be covered, even though they are known in advance.
As described, one can end up with a number of different means to determine flexibility requirements. For example, for large changes in net load, it may be more straightforward to determine the overall net load variability, by time of day or year. This can be compared to available flexibility, as described later, to determine whether flexibility is sufficient. On the other hand, using specific wind, solar and load characteristics can give a more accurate representation of what the specific needs at a given time interval may be, particularly when examining these issues in operations or operational simulations. These can then be combined, assuming no correlation, using a geometric sum, which is the square root of sum of squares (it is not recommended to just add wind, solar and load together directly as this would overestimate requirements). The InFLEXion tool currently has capability to determine ramping requirements for each individual source (wind, solar, load) separately, or as a combined net load variability. Both are recommended to be examined, with the user determining flexibility needs based on individual components or combined net load based on their specific results and system.
References and Footnotes
- ↑ 1.0 1.1 1.2 1.3 Power System Flexibility Metrics EPRI, Palo Alto, CA: 2013. 3002000331
- ↑ Flexible and Strategic Transmission Planning. EPRI, Palo Alto, CA: 2011. 1021931
- ↑ Metrics and Methods for Consideration of Flexibility in Power System Planning. EPRI, Palo Alto, CA: 2012. 1024274
- ↑ 4.0 4.1 Flexibility in Transmission and Resource Planning. EPRI, Palo Alto, CA: 2014. 3002003581
- ↑ 5.0 5.1 System Flexibility Assessment and Analysis. EPRI, Palo Alto, CA: 2015. 3002005763.
- ↑ Strategic and Flexible System Planning – 2016 Update: Deliverable Flexibility and Flexibility in Resource Adequacy. EPRI, Palo Alto, CA: 2016. 3002008379.
- ↑ Metrics for Quantifying Flexibility in Power System Planning, EPRI, Palo Alto, CA: 2014. 300200424
- ↑ Southwest Power Pool, 2016 Wind Integration Study, available https://www.spp.org/Documents/34200/2016%20Wind%20Integration%20Study%20(WIS)%20Final.pdf, 2016
- ↑ J. Hargreaves, E. Hart, R. Jones, A. Olsen, REFLEX: An Adapted Production Simulation Methodology for Flexible Capacity Planning, accessed Nov 2016 at www.ethree.com
- ↑ Pacific Gas and Electric, Collaborative Review of Collaborative Review of Planning Models, April 2014.
- ↑ More details on this project and the associated tool can be found at http://irena.org/publications/2018/Nov/Power-system-flexibility-for-the-energy-transition
- ↑ 12.0 12.1 A. Mills, J. Seel, Flexibility Inventory for Western Resource Planners, LBNL report, available http://emp.lbl.gov/publications/flexibility-inventory-western
- ↑ IRENA (2018), Power System Flexibility for the Energy Transition, Part 1: Overview for policy makers, International Renewable Energy Agency, Abu Dhabi
- ↑ International Energy Agency, The Power of Transformation - Wind, Sun and the Economics of Flexible Power Systems. Published 2014, available www.iea.org